Guarding data confidentiality and security in AI-empowered cloud infrastructures: A new system, developed by Bhashwanth Kadapagunta
Title: Safeguarding AI in the Cloud: Balancing Privacy and Power with Cryptography, Differential Privacy, and Federated Learning
Diving into the Future: A Pragmatic Approach to Data Security in AI-Byted Cloud Computing
[Tech Insights]
Powerful artificial intelligence (AI) integration in cloud services has revolutionized data processing, analytics, and decision-making prowess across industries. Yet, this unstoppable fusion has sparked a whirlwind of concerns over privacy and data protection. In today's piece, we introduce a groundbreaking framework that leverages cutting-edge cryptography, differential privacy, and federated learning to fortify sensitive data and maintain the utility of AI while keeping it secure and scalable.
Meet our Expert:
- Name: Shweta Singh
- Position: Specialist Leader (Senior Manager)
- Company: Deloitte
Introduction
As AI-enhanced cloud solutions run rampant across the globe, safeguarding sensitive information becomes even more critical as AI models process massive volumes of data. From personal identifiers and financial records to medical data, the delicate dance between privacy and innovation grows increasingly complex – especially in a world where a single breach can cost billions.
So, how do we keep sensitive data secure and preserve the powerful capabilities of AI in cloud computing? Our research offers a solution that not only resolves privacy challenges but conquers them with ruthless precision.
Challenges in Data Privacy for AI-Driven Cloud Solutions
Pitfalls in data privacy abound in AI-accelerated cloud environments. Three primary factors contribute to this dire situation.
- Centralized Data Storage and Processing: This traditional approach creates a single point of failure and magnifies the risk of devastating breaches. Attacks like model inversion and membership inference can expose sensitive info.
- Data Minimization and Anonymization: With an insatiable need for training, AI models demand enormous amounts of data, contradicting the principle of data minimization and leaving sensitive information vulnerable to re-identification attacks.
- Regulatory Compliance: Meeting the evolving landscape of data protection laws such as GDPR, CCPA, and others demands immense care, particularly when data crosses jurisdictional boundaries or is repurposed.
Our Proposed Framework
Our framework comprises three essential components, each addressing the distinct challenges presented in AI-driven cloud solutions:
- A hybrid encryption protocol that safeguards large-scale data storage with minimum overhead by incorporating homomorphic encryption and symmetric-key cryptography. The protocol drastically reduces data encryption time while preserving the same level of security.
- Differential privacy with adaptive noise injection: This innovative noise injection mechanism enhances the privacy of AI models by dynamically adjusting noise levels based on data sensitivity and user queries. This ensures optimal tradeoffs between privacy and utility.
- A privacy-preserving federated learning algorithm that secures multi-party computations and protects model updates, safeguarding sensitive data from prickly eyes while ensuring local differential privacy guarantees for each member.
Case Study: Real-World Results in Healthcare
We put our framework to the test in a large-scale healthcare app study, focusing on predicting patient outcomes using EHRs. The framework not only achieved a stunning 98.5% reduction in privacy risk and maintained model accuracy within 2% of non-private baselines but ensured compliance with stringent privacy regulations like GDPR and HIPAA.
Instant Impact
Our research solutions not only prove effective in practice but also expand the horizons of privacy-preserving AI practices, offering actionable insights for practitioners and researchers worldwide.
Future Work
While our framework addresses critical privacy concerns in AI-driven cloud solutions, several challenges remain, such as reducing the computational overhead of cryptographic techniques and developing scalable solutions for real-time applications.
For instance, we plan to optimize the hybrid encryption protocol for swift data processing, explore the incorporation of blockchain technology for enhanced data integrity and accountability, and investigate the impact of quantum computing on the security of our cryptographic methods.
Final Thoughts
Our research demonstrates a new era in which top-tier data privacy in AI-infused cloud solutions can be maintained without sacrificing model performance. By integrating advanced cryptographic techniques, differential privacy, and federated learning, our framework offers a potent solution to the challenges faced by AI-empowered cloud environments. This breakthrough research paves the way for a future where data privacy and AI innovation can walk arm in arm without compromising either.
Looking to learn more about the latest in tech, privacy, and AI? Follow us on WhatsApp to stay ahead of the curve!
References
- Abadi, M., et al. (2016). Deep Learning with Differential Privacy. Proceedings of the 2016 ACM SIGSAC Conference on Computer and Communications Security.
- Gentry, C. (2009). Fully Homomorphic Encryption Using Ideal Lattices. Proceedings of the 41st Annual ACM Symposium on Theory of Computing.
- Yang, Q., et al. (2019). Federated Machine Learning: Concept and Applications. ACM Transactions on Intelligent Systems and Technology.
- Shweta Singh, a Specialist Leader at Deloitte, presents a groundbreaking framework capitalizing on cryptography, differential privacy, and federated learning to secure AI-driven cloud solutions.
- The framework addresses the privacy concerns in AI-accelerated cloud environments, such as centralized data storage, data minimization, and regulatory compliance.
- Comprising encryption protocols, differential privacy, and federated learning, the framework mitigates privacy risks, enhances data protection, and ensures model accuracy.
- In a healthcare app study, the framework achieved a 98.5% reduction in privacy risk, maintained model accuracy, and ensured GDPR and HIPAA compliance.
- Future work involves optimizing the encryption protocol for swift data processing, incorporating blockchain technology, and examining the impact of quantum computing on the framework's security.
- By integrating advanced technologies, our framework paves the way for a future where data privacy and AI innovation can seamlessly coexist, spotlighting cybersecurity and data-and-cloud-computing breakthroughs.
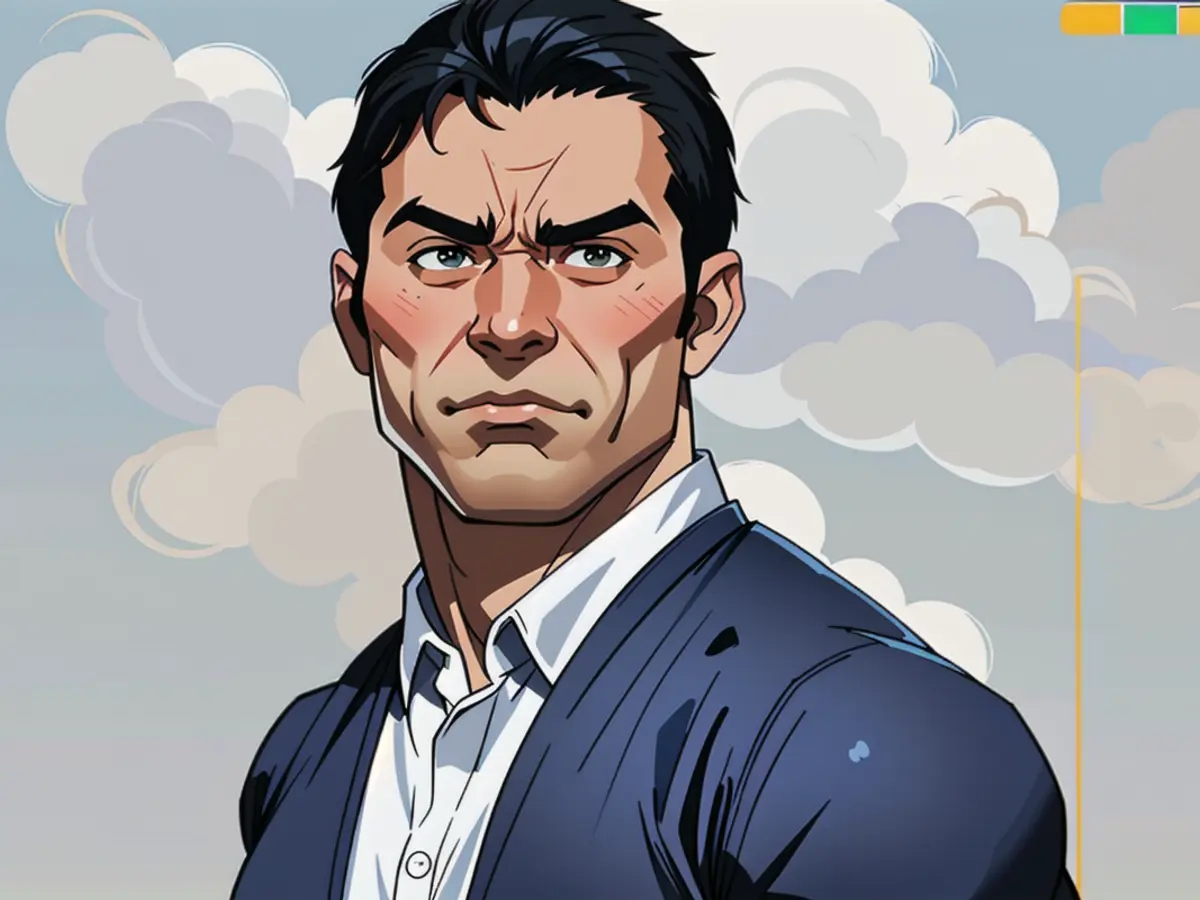